Vahid Balazadeh
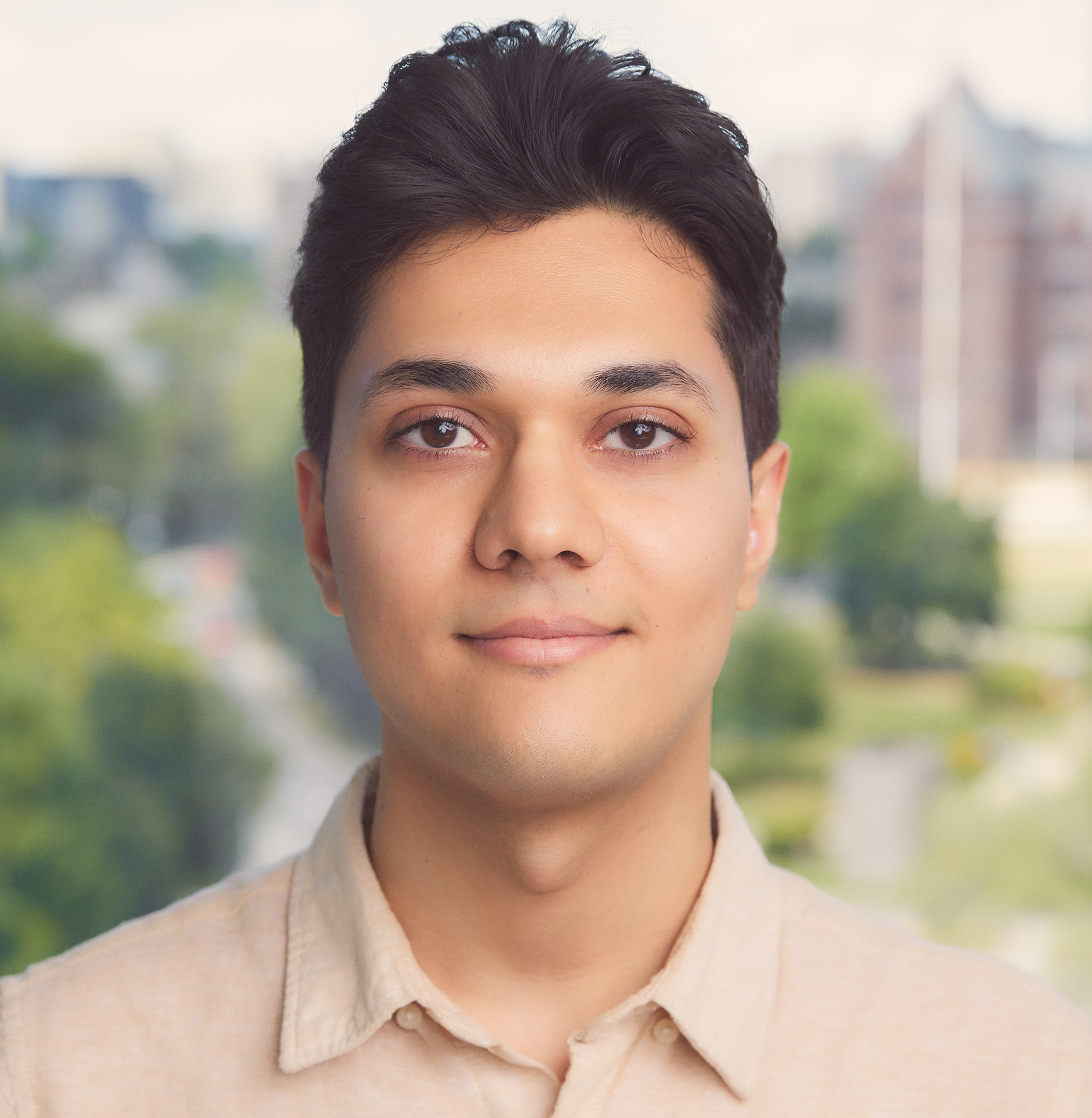
I’m a Ph.D. student in Computer Science at the University of Toronto, supervised by Rahul G. Krishnan. I’m also fortunate to have Vasilis Syrgkanis from Stanford University as my external advisor. Before starting my Ph.D., I was a research intern at MPI-SWS, where I had the opportunity to work with Manuel Gomez Rodriguez on human-machine collaboration in reinforcement learning. I hold a double major B.Sc. in Computer Engineering and Mathematics from the Sharif University of Technology.
My research focus lies in understanding the concepts and mechanisms that aid humans in optimal decision-making, particularly in applications where simulation is costly or infeasible, such as healthcare. To this end, I’m working on causal inference from observational data and its intersection with machine learning, as well as imitation learning and reinforcement learning from offline observations.
One particular direction I’m excited about is leveraging existing observational data and synthetic data generation to develop data-driven algorithms for causal decision-making, applicable in realistic settings such as partial observability. I explore an aspect of this idea in a recent paper titled “Sequential Decision Making with Expert Demonstrations under Unobserved Heterogeneity”. Additionally, I’ve been exploring the in-context learning capabilities of transformer-based architectures to advance the design of such data-driven algorithms. We discuss an application of this idea for personalized adaptation in “Personalized Adaptation via In-Context Preference Learning”. In the past, I have worked on using generative models for causal effect estimation, causal structure learning from observational data, and human-centric reinforcement learning. For more information, please see my Research section.
Experience
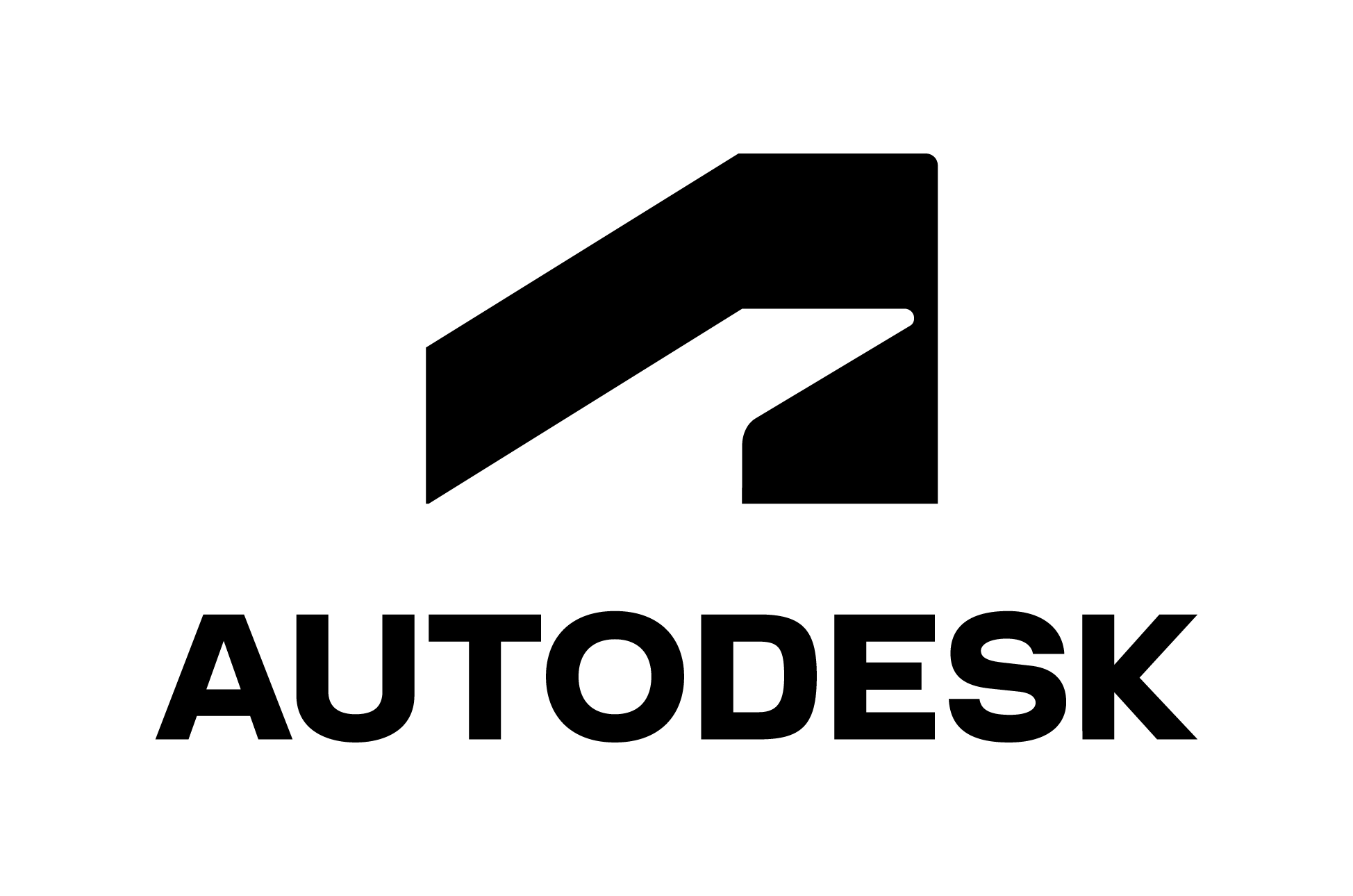
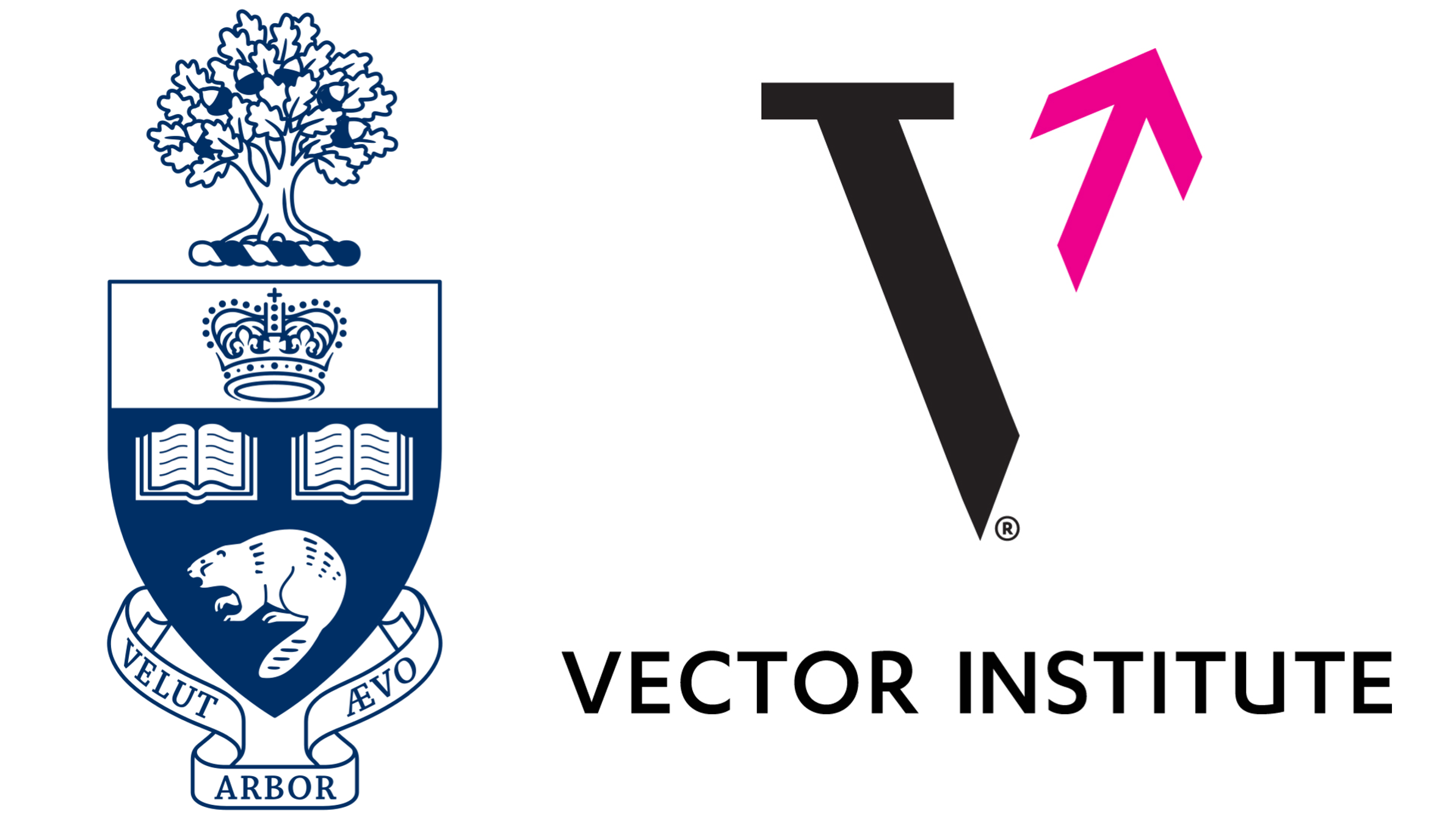
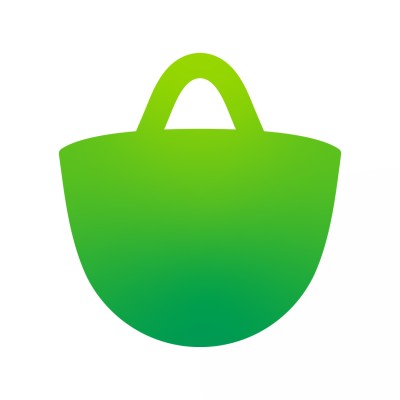
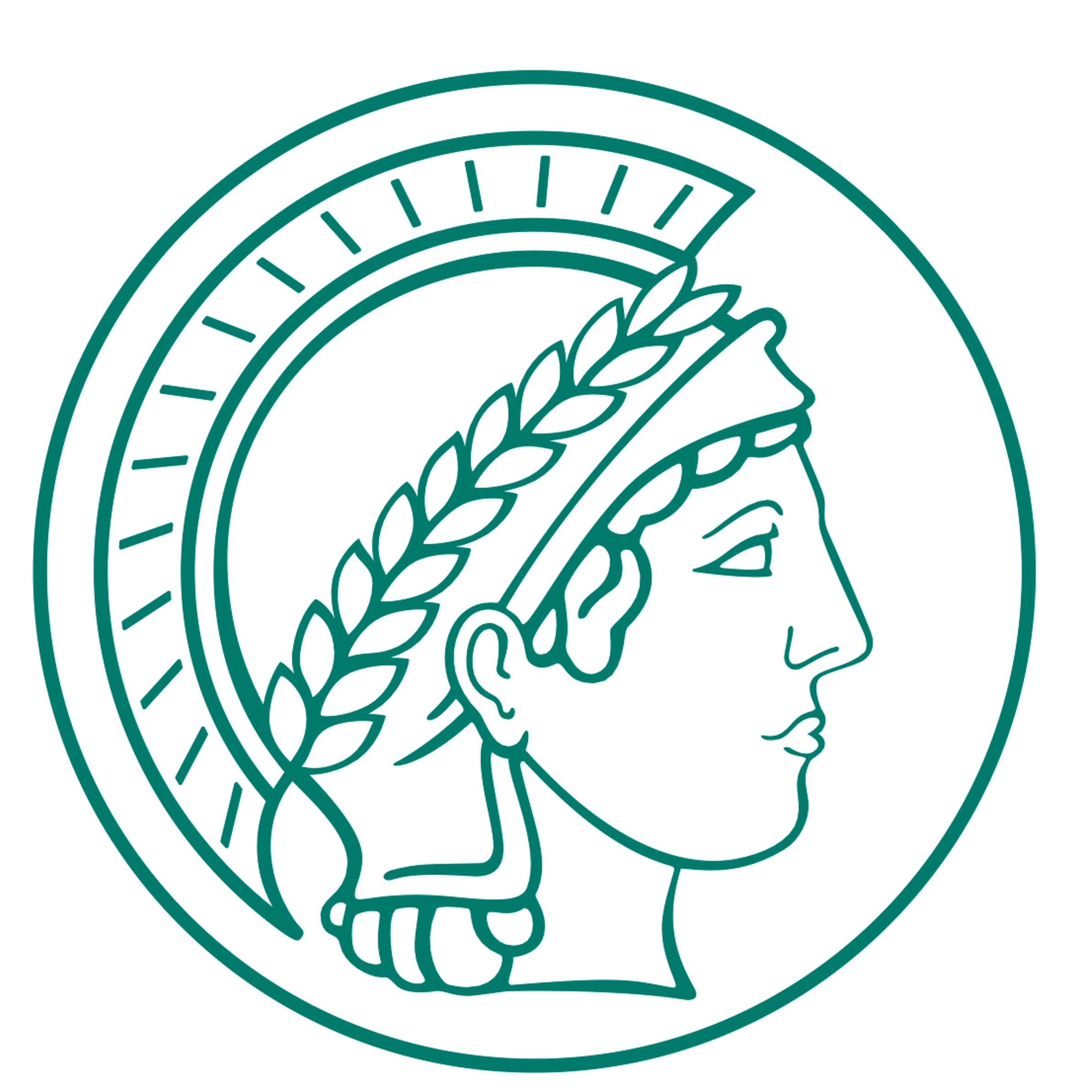
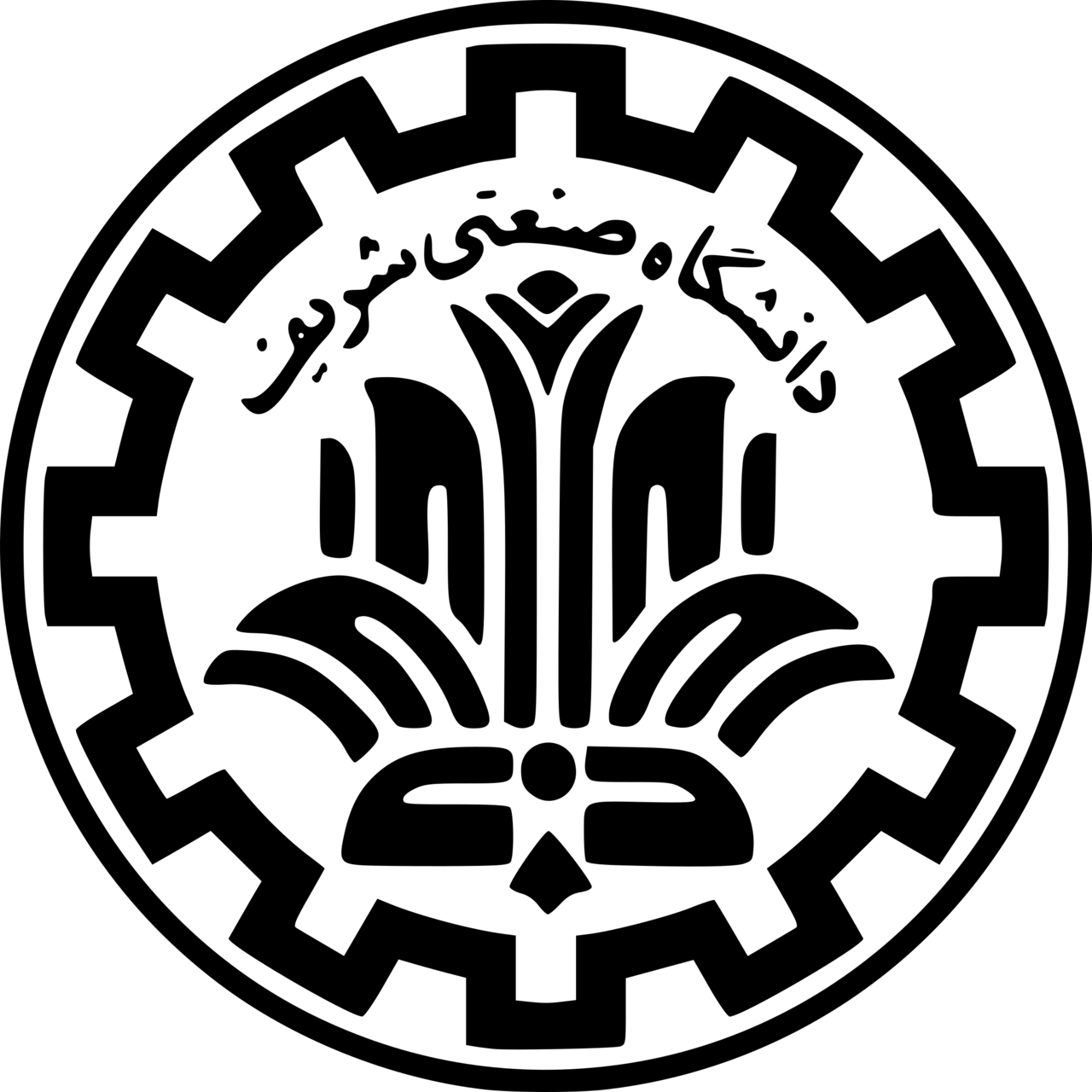